MQ-Dash: A novel prescriptive digital dashboard for aged care - a journey from inception to evaluation
Tracks
Chancellor 6
Best practice
Dementia
Design
Education and Training
Evidence based practice
Falls / Fall Preventaion
Future Directions
Health Management
Implementation
Innovation
Literature
Medications
Non-pharmacological interventions
Quality improvement
Residential
Technology
Wellness / Well Being
Thursday, November 14, 2024 |
4:00 PM - 5:00 PM |
Speaker
Dr Amy Nguyen
Research Fellow
Australian Institute Of Health Innovation, Macquarie University
MQ-Dash: A novel prescriptive digital dashboard for aged care - a journey from inception to evaluation
Abstract
Background:
This symposium explores findings arising from 'MQ-Dash' – a predictive falls and wellbeing dashboard that provides personalised recommendations to improve care – currently trialled in residential aged care. The stages of idea inception, dashboard co-design, development of the infrastructure and functionalities, dashboard implementation, evaluating its use, and future directions will be discussed. Insights garnered through extensive collaboration with aged care users, healthcare providers, executives, industry, and governing bodies, will be presented from technical and translation perspectives.
Aims:
To provide real-world learnings to support those co-designing and implementing a digital platform with end-users in aged care.
Overview of symposium activities:
1. Overview - Introduction to symposium, aims and objectives of 'MQ-Dash' project.
2. Co-design of dashboard with end-users – Active engagement with stakeholders to ensure adequate collaboration, and how to incorporate stakeholders' feedback.
3. Development of dashboard infrastructure – Translation of stakeholders’ co-design preferences into building dashboard’s sustainable architecture; and development and integration of dynamic falls predictive tool and personalised falls prevention interventions.
4. Dashboard implementation – Strategies to implement MQ-Dash into practice across residential aged care facilities to ensure uptake and usability.
5. Evaluation of dashboard – Assessing clinical, process and economic outcomes of MQ-Dash.
6. Future of dashboards – Digital health in the future of aged care.
7. Q&A with audience
Audience learning outcomes:
How to:
-Actively collaborate with aged care stakeholders
-Build sustainable health technology infrastructures
-Design implementation plans for optimal use of health technologies
-Evaluate project outcomes
-Incorporate digital health into aged care research and practice
This symposium explores findings arising from 'MQ-Dash' – a predictive falls and wellbeing dashboard that provides personalised recommendations to improve care – currently trialled in residential aged care. The stages of idea inception, dashboard co-design, development of the infrastructure and functionalities, dashboard implementation, evaluating its use, and future directions will be discussed. Insights garnered through extensive collaboration with aged care users, healthcare providers, executives, industry, and governing bodies, will be presented from technical and translation perspectives.
Aims:
To provide real-world learnings to support those co-designing and implementing a digital platform with end-users in aged care.
Overview of symposium activities:
1. Overview - Introduction to symposium, aims and objectives of 'MQ-Dash' project.
2. Co-design of dashboard with end-users – Active engagement with stakeholders to ensure adequate collaboration, and how to incorporate stakeholders' feedback.
3. Development of dashboard infrastructure – Translation of stakeholders’ co-design preferences into building dashboard’s sustainable architecture; and development and integration of dynamic falls predictive tool and personalised falls prevention interventions.
4. Dashboard implementation – Strategies to implement MQ-Dash into practice across residential aged care facilities to ensure uptake and usability.
5. Evaluation of dashboard – Assessing clinical, process and economic outcomes of MQ-Dash.
6. Future of dashboards – Digital health in the future of aged care.
7. Q&A with audience
Audience learning outcomes:
How to:
-Actively collaborate with aged care stakeholders
-Build sustainable health technology infrastructures
-Design implementation plans for optimal use of health technologies
-Evaluate project outcomes
-Incorporate digital health into aged care research and practice
Biography
Dr Amy Nguyen is a digital health researcher whose research focuses on implementation of various health technologies in the aged care, general practice and chronic disease sectors. Dr Nguyen leads work focusing on the co-design, usability, feasibility and quality of health technologies in the delivery of safe healthcare, using a myriad of qualitative and quantitative methods. Amy has worked on the co-design of health technologies such as smartphone apps, prescribing software and electronic clinical dashboards in collaboration with patients, clinicians, allied health, management and policymakers. Dr Nguyen’s work has guided decisions in the national development and implementation of My Health Record in telehealth, aged care and specialist sectors. Amy also has a keen interest in effective scientific communication, and has been an active STEM Mentor for The New York Academy of Sciences since 2016. Dr Nguyen is a Digital Health Advisor for the Australian Digital Health Agency, providing expert knowledge to the design, implementation and evaluation of digital health initiatives across the Australian healthcare system. She also is an Academic Advisor on the National Digital Medicines Advisory Group.
Dr Amy Nguyen
Research Fellow
Australian Institute Of Health Innovation, Macquarie University
Aged care consumers’ and healthcare providers' digital dashboard design preferences
Abstract
Introduction:
The relevance, extent and presentation of information displayed in residential aged care clinical dashboards to support timely, person-centred care, remains unclear, Therefore, to inform the design of a digital dashboard, sequential qualitative studies were conducted to understand what and how information is needed to be presented from the perspective of 1) aged care consumers and 2) aged care healthcare providers.
Methods:
First, semi-structured interviews, exploring consumer information needs and how this information should be presented in a dashboard, were conducted with aged care consumers (n=26) and general practitioners (n=3). Interview findings informed the development of a dashboard prototype. Second, feedback was received for the dashboard prototypes in eight workshops (n=20 aged care healthcare providers). Interview and workshop transcripts were analysed using an inductive content or template analysis approach.
Results:
Aged care consumers said information regarding medications, medical conditions and an overview of the resident were important to them. They stated this information should be presented on a dashboard using large text, colours, graphs, and with customisable functionalities. Aged care healthcare providers provided content, functionality and design feedback on the dashboard including presentation of resident medication data, benchmarking, filtering and drilling down functionalities, evidence-based decision support, and minimal alerts.
Conclusion:
A predictive dashboard for falls and wellbeing in aged care was developed iteratively with consultation from aged care stakeholders. The dashboard contents and functionalities were based on the preferences of end-users. These preferences may be relevant and translated to the development of other digital platforms in aged care.
The relevance, extent and presentation of information displayed in residential aged care clinical dashboards to support timely, person-centred care, remains unclear, Therefore, to inform the design of a digital dashboard, sequential qualitative studies were conducted to understand what and how information is needed to be presented from the perspective of 1) aged care consumers and 2) aged care healthcare providers.
Methods:
First, semi-structured interviews, exploring consumer information needs and how this information should be presented in a dashboard, were conducted with aged care consumers (n=26) and general practitioners (n=3). Interview findings informed the development of a dashboard prototype. Second, feedback was received for the dashboard prototypes in eight workshops (n=20 aged care healthcare providers). Interview and workshop transcripts were analysed using an inductive content or template analysis approach.
Results:
Aged care consumers said information regarding medications, medical conditions and an overview of the resident were important to them. They stated this information should be presented on a dashboard using large text, colours, graphs, and with customisable functionalities. Aged care healthcare providers provided content, functionality and design feedback on the dashboard including presentation of resident medication data, benchmarking, filtering and drilling down functionalities, evidence-based decision support, and minimal alerts.
Conclusion:
A predictive dashboard for falls and wellbeing in aged care was developed iteratively with consultation from aged care stakeholders. The dashboard contents and functionalities were based on the preferences of end-users. These preferences may be relevant and translated to the development of other digital platforms in aged care.
Biography
Dr Amy Nguyen is a digital health researcher whose research focuses on implementation of various health technologies in the aged care, general practice and chronic disease sectors. Dr Nguyen leads work focusing on the co-design, usability, feasibility and quality of health technologies in the delivery of safe healthcare, using a myriad of qualitative and quantitative methods. Amy has worked on the co-design of health technologies such as smartphone apps, prescribing software and electronic clinical dashboards in collaboration with patients, clinicians, allied health, management and policymakers. Dr Nguyen’s work has guided decisions in the national development and implementation of My Health Record in telehealth, aged care and specialist sectors. Amy also has a keen interest in effective scientific communication, and has been an active STEM Mentor for The New York Academy of Sciences since 2016. Dr Nguyen is a Digital Health Advisor for the Australian Digital Health Agency, providing expert knowledge to the design, implementation and evaluation of digital health initiatives across the Australian healthcare system. She also is an Academic Advisor on the National Digital Medicines Advisory Group.
Dr Nasir Wabe
Senior Research Fellow
Australian Institute Of Health Innovation, Macquarie University
FRIPAC: A dynamic tool for predicting and monitoring fall risk in residential aged care
Abstract
Background: Falls pose a significant challenge in residential aged care (RAC), necessitating ongoing risk assessment for effective prevention. We aimed to develop and internally validate a dynamic fall risk prediction and monitoring tool for aged care (FRIPAC) and to create separate point-based scoring systems by dementia status.
Method: A longitudinal cohort study using 8-year electronic data (2014-2022) from 27 RAC homes (n=5492 residents) in Sydney, Australia. The outcome was the incidence of falls. We used landmarking dynamic prediction for model development, a time-dependent area under the curve (AUC) for evaluations, and a regression coefficient approach to create point-based scoring systems.
Results: The falls incident rate was 7.76 falls/1000 resident-day. The model identified 15 predictors of falls in dementia and 12 in non-dementia cohorts. Falls history was the key predictor of subsequent falls in dementia (Hazard Ratio 4.75, 95% CI 4.45-5.06) and non-dementia cohorts (Hazard Ratio 4.20, 95% CI 3.87-4.57). The use of drugs for bone disease was the only protective factor against falls, particularly in the dementia cohort. The AUC ranged from 0.67-0.87 for dementia and from 0.66-0.86 for non-dementia cohorts but generally remained between 0.75-0.85. The total point risk score ranged from -2 to 57 for dementia and 0 to 55 for non-dementia cohorts.
Conclusion: Our innovative risk prediction models and scoring systems offer timely, person-centred information for ongoing prediction and monitoring of fall risk in RAC homes. Integrated into the ‘MQ-Dash’ dashboard, our tool serves as a decision support system, empowering targeted proactive interventions to prevent falls.
Method: A longitudinal cohort study using 8-year electronic data (2014-2022) from 27 RAC homes (n=5492 residents) in Sydney, Australia. The outcome was the incidence of falls. We used landmarking dynamic prediction for model development, a time-dependent area under the curve (AUC) for evaluations, and a regression coefficient approach to create point-based scoring systems.
Results: The falls incident rate was 7.76 falls/1000 resident-day. The model identified 15 predictors of falls in dementia and 12 in non-dementia cohorts. Falls history was the key predictor of subsequent falls in dementia (Hazard Ratio 4.75, 95% CI 4.45-5.06) and non-dementia cohorts (Hazard Ratio 4.20, 95% CI 3.87-4.57). The use of drugs for bone disease was the only protective factor against falls, particularly in the dementia cohort. The AUC ranged from 0.67-0.87 for dementia and from 0.66-0.86 for non-dementia cohorts but generally remained between 0.75-0.85. The total point risk score ranged from -2 to 57 for dementia and 0 to 55 for non-dementia cohorts.
Conclusion: Our innovative risk prediction models and scoring systems offer timely, person-centred information for ongoing prediction and monitoring of fall risk in RAC homes. Integrated into the ‘MQ-Dash’ dashboard, our tool serves as a decision support system, empowering targeted proactive interventions to prevent falls.
Biography
Dr Nasir Wabe is a Senior Research Fellow at the Australian Institute of Health Innovation, Macquarie University, with a multi-disciplinary background (pharmacy, epidemiology, data science). He has expertise in the areas of outcome-based health services research, health informatics, pharmacoepidemiology and quality use of medicine. Dr Wabe has extensive experience in conducting data linkage and analysis of big health data obtained from primary care, hospitals, and residential aged care. Dr Wabe has a strong track record of research outputs including >60 peer-reviewed articles (h-index 21, i-10 27). He has been successful in securing >$6.5 million in research funding.
Dr Sandun Malpriya Silva
Research Fellow
Australian Institute Of Health Innovation, Macquarie University
Development of a falls prevention dashboard in residential aged care: A predictive dashboard with prescriptive decision support
Abstract
Background: Falls pose a significant health risk for older individuals residing in residential aged care facilities (RACFs). Current fall prevention methods fail to offer dynamic risk predictions due to the failure to access contemporary and longitudinal data. To address this, we have developed 'MQ-Dash,' a dynamic predictive analytics dashboard utilising routinely collected data aimed at dynamically identifying residents at increased risk of falls.
Method: This study was conducted in collaboration with a major aged care provider in NSW. Data from resident profiles, medications, falls incidents, and risk assessments were used. MQ-Dash was co-designed through an iterative process, involving data extraction, model schema development, and visualisation stages. A dynamic falls risk predictive and monitoring tool was developed using a landmarking approach. The dashboard designed to mitigate the impact of underlying data system changes. This approach aims to enhance care by providing timely risk ratings and resident information while ensuring resilience to data system alterations.
Result: The system was developed using Power BI and R programming, by connecting the data silos to generate insights for informed decision-making. The dashboard includes facility-level reports and resident-level reports which provide facility and resident specific risk ratings and fall profiles. Resident reports provide dynamic falls prediction, real-time monitoring of falls risk factors, and timely evidence-based personalised recommendations to prevent falls.
Conclusion: This study describes the technical processes needed to develop the backend and front user interface of a predictive dashboard, highlighting the value of building a sustainable and transferable dashboard architecture for use in aged care.
Method: This study was conducted in collaboration with a major aged care provider in NSW. Data from resident profiles, medications, falls incidents, and risk assessments were used. MQ-Dash was co-designed through an iterative process, involving data extraction, model schema development, and visualisation stages. A dynamic falls risk predictive and monitoring tool was developed using a landmarking approach. The dashboard designed to mitigate the impact of underlying data system changes. This approach aims to enhance care by providing timely risk ratings and resident information while ensuring resilience to data system alterations.
Result: The system was developed using Power BI and R programming, by connecting the data silos to generate insights for informed decision-making. The dashboard includes facility-level reports and resident-level reports which provide facility and resident specific risk ratings and fall profiles. Resident reports provide dynamic falls prediction, real-time monitoring of falls risk factors, and timely evidence-based personalised recommendations to prevent falls.
Conclusion: This study describes the technical processes needed to develop the backend and front user interface of a predictive dashboard, highlighting the value of building a sustainable and transferable dashboard architecture for use in aged care.
Biography
Dr. S. Sandun Malpriya Silva is a statistician specialized in biostatistics and data science.
He is currently working as a research fellow at Australian Institute of Health Innovation (AIHI). He has worked as a biostatistician at National Health Medical Research Council (NHMRC) Clinical Trial Center, University of Sydney, prior joining to AIHI.
He has completed his bachelor’s in Statistics at University of Colombo, Sri Lanka. After a year of working as a data analyst/scientist in Sri Lanka, he was offered a full scholarship from Department of Health Science and Biostatistics at Swinburne University of Technology Australia to follow a PhD in Statistics (STA90001) in the scope of Health Science (DR-HTHSCI).
He has also worked with Peter MacCallum Cancer Research Centre, Melbourne on various studies to solve real world problems. The findings of these studies have been published in leading academic journals.
Dr Andrea Timothy
Research Fellow
Australian Institute of Health Innovation, Macquarie University
Implementing a predictive falls and well-being dashboard in residential aged care
Abstract
MQ-Dash is a predictive analytics dashboard that utilises routinely collected data relating to falls, quality of life, and quality of care experience to predict the risk of poor outcomes, and provide evidence-based decision support to monitor and manage resident care. Our objectives were to implement, test, and evaluate MQ-Dash in residential aged care facilities (RACFs).
The Consolidated Framework for Implementation Research (CFIR) was used to guide the implementation and evaluation of MQ-Dash in this setting. Components of the MQ-Dash project were mapped to CFIR domains, and a pre-implementation assessment was conducted to 1) understand the environment that MQ-Dash was to be implemented into, 2) identify barriers and facilitators to implementation, and 3) identify strategies to facilitate the implementation process.
Pre-implementation assessment findings were used to develop an implementation plan summarising tasks and strategies relating to Capacity Building, Collaboration, Communication and Engagement, and Adaptation to progress implementation of MQ-Dash at RACFs.
MQ-Dash is currently being trialled in RACFs. Ongoing evaluation of MQ-Dash incorporates usability testing and implementation process evaluation via surveys and interviews with end-users, as well as regular progress meetings with Project Champions during the trial period. Findings are collated in a lightning report to 1) share with implementation team members, and 2) identify if any rapid adaptations need to be made either to the intervention, or the implementation process.
This study describes the implementation process, lessons learnt during MQ-Dash pilot testing and early stages of the randomised control trial, and provides a model for other intervention implementation in RACFs.
The Consolidated Framework for Implementation Research (CFIR) was used to guide the implementation and evaluation of MQ-Dash in this setting. Components of the MQ-Dash project were mapped to CFIR domains, and a pre-implementation assessment was conducted to 1) understand the environment that MQ-Dash was to be implemented into, 2) identify barriers and facilitators to implementation, and 3) identify strategies to facilitate the implementation process.
Pre-implementation assessment findings were used to develop an implementation plan summarising tasks and strategies relating to Capacity Building, Collaboration, Communication and Engagement, and Adaptation to progress implementation of MQ-Dash at RACFs.
MQ-Dash is currently being trialled in RACFs. Ongoing evaluation of MQ-Dash incorporates usability testing and implementation process evaluation via surveys and interviews with end-users, as well as regular progress meetings with Project Champions during the trial period. Findings are collated in a lightning report to 1) share with implementation team members, and 2) identify if any rapid adaptations need to be made either to the intervention, or the implementation process.
This study describes the implementation process, lessons learnt during MQ-Dash pilot testing and early stages of the randomised control trial, and provides a model for other intervention implementation in RACFs.
Biography
Dr Andrea Timothy is a Research Fellow at the Australian Institute of Health Innovation, Macquarie University. She has a background in science and public health, with a specific focus on health systems strengthening and implementation science. Andrea currently works across multiple projects focusing on developing and implementing approaches to improve aged care medication challenges in collaboration with aged care providers, clinicians and policy-makers.
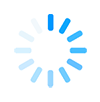